😊 About Me
My name is Kaining Zhang, a Ph.D. student at Wuhan University. My research interests mainly lie in the intersection between 3D geometry and deep learning, specifically for tasks such as image matching, large-scale visual localization, 3D scene reconstruction, etc. Currently I am looking for a postdoctoral position to continue my research in related fields.
📖 Educations
- 2023.12 - 2025.01, visiting Ph.D. student, Computer Vision Group, University of Bern, Switzerland.
- Advisor: Prof. Paolo Favaro
- 2019.09 - 2024.06 (expected), Ph.D. student, Multi-Spectral Vision Processing Lab, Wuhan University, Wuhan, China.
- Advisor: Prof. Jiayi Ma
- 2015.09 - 2019.06, B.Sc. in Electronic Information Science and Technology, Electronic Information School, Wuhan University, Wuhan, China.
📝 Publications
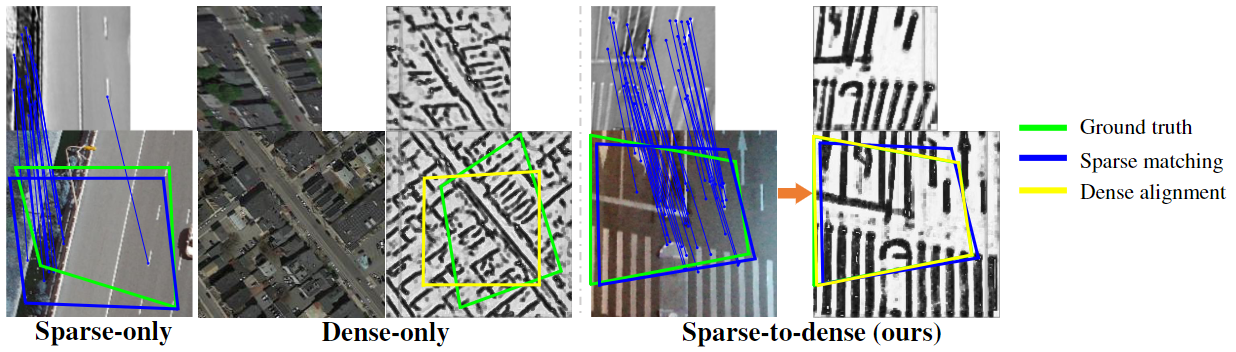
Sparse-to-dense Multimodal Image Registration via Multi-Task Learning
Kaining Zhang, Jiayi Ma
International Conference on Machine Learning (ICML), 2024
- We propose a novel matching paradigm to address the drawbacks that current multimodal image matching paradigms are facing. Experiments are conducted on several cross-modal scenarios, including visible-infrared, visible-near infrared, and GoogleEarth.
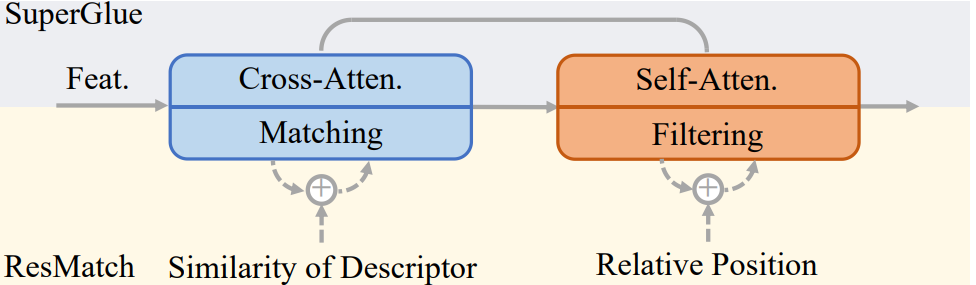
ResMatch: Residual Attention Learning for Feature Matching
Yuxin Deng, Kaining Zhang, Zizhuo Li, Shihua Zhang, Yansheng Li, Jiayi Ma
Proceedings of the AAAI Conference on Artificial Intelligence (AAAI), 2024
- We take a deep look at the attention mechanism of SuperGlue and rethink cross-attention and self-attention from the perspective of traditional feature matching and filtering. Our well-designed attention approach leads to significant performance improvements on several benchmarks.
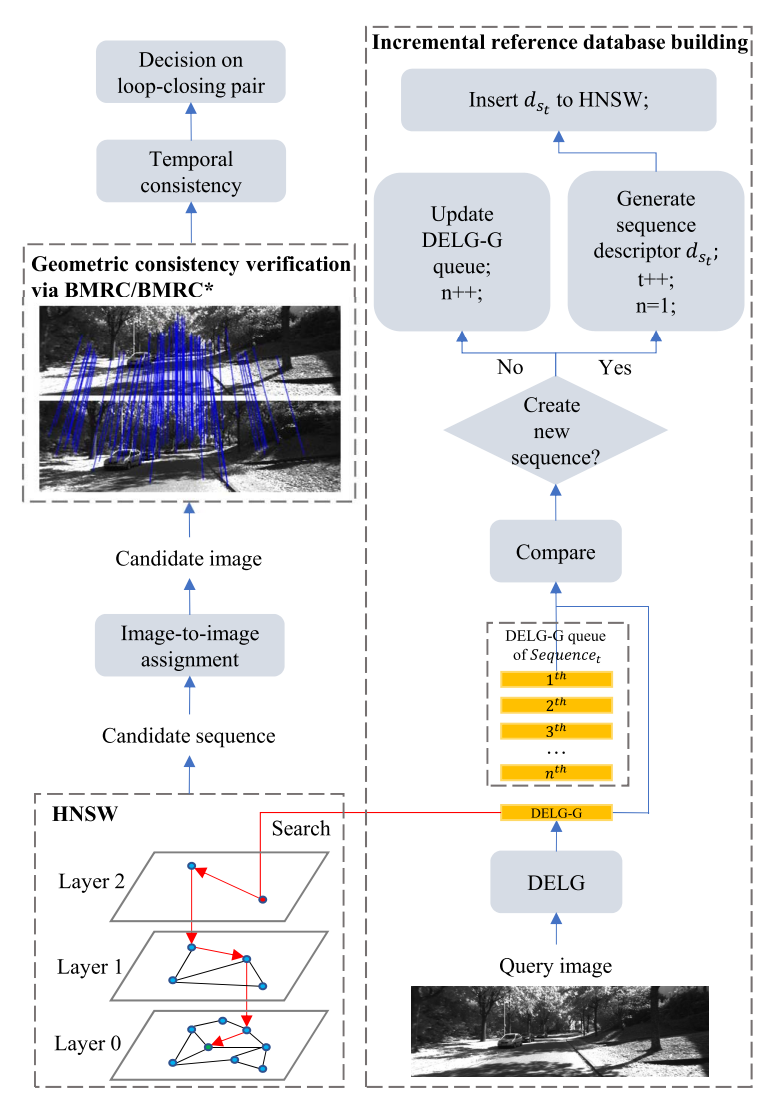
Loop Closure Detection via Bidirectional Manifold Representation Consensus
Kaining Zhang, Zizhuo Li, Jiayi Ma
IEEE Transactions on Intelligent Transportation Systems (TITS), 2022
- This is a comprehensive extension of our ICRA 2021. We speed up our algorithm by 63% and build the incremental database via HNSW. Meanwhile, experiments are conducted on more challenging datasets.
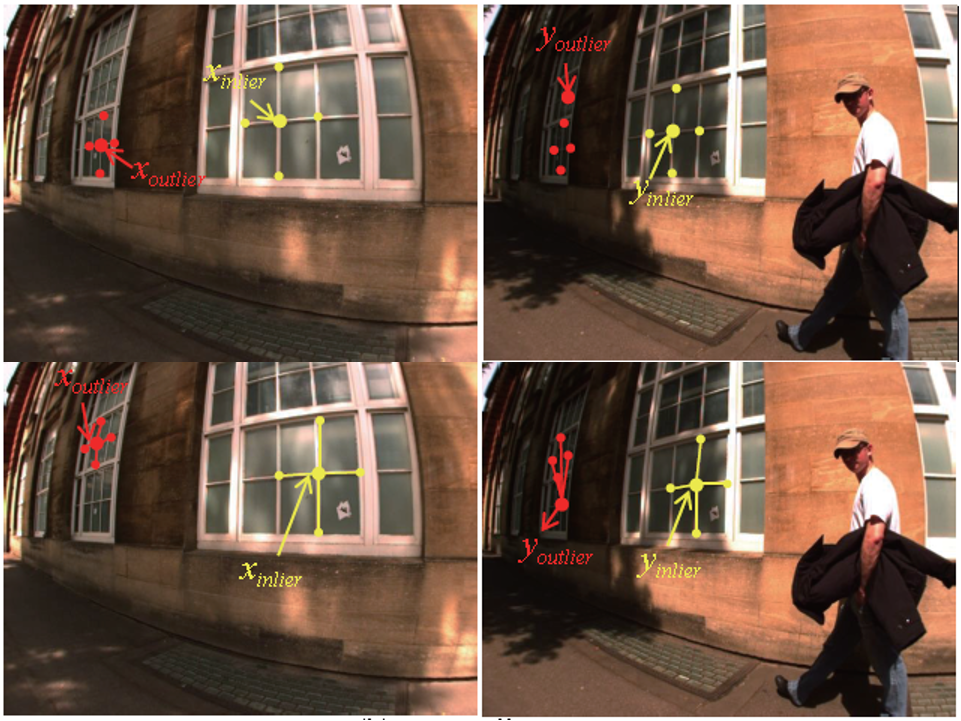
Loop Closure Detection with Reweighting NetVLAD and Local Motion and Structure Consensus
Kaining Zhang, Jiayi Ma, Junjun Jiang
IEEE/CAA Journal of Automatica Sinica (JAS), 2022
- We propose AttentionNetVLAD to extract more powerful image representation, and address feature matching via information lying on both a 2D space and an intrinsic manifold.
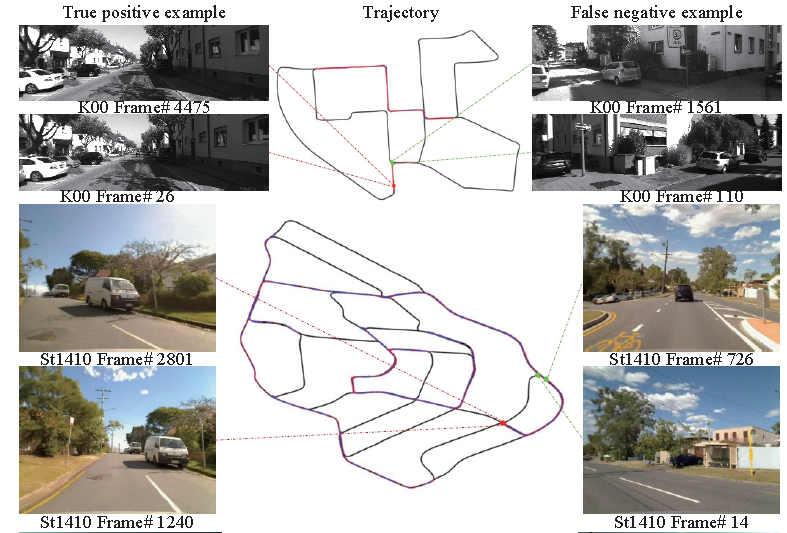
Loop Closure Detection via Locality Preserving Matching with Global Consensus
Jiayi Ma, Kaining Zhang, Junjun Jiang
IEEE/CAA Journal of Automatica Sinica (JAS), 2022
- A simple yet surprisingly effective feature matching approach is proposed for scenes within loop closure detection tasks (e.g., scenes with repetitive structures). The algorithm can provide reliable correspondences within only a few milliseconds.
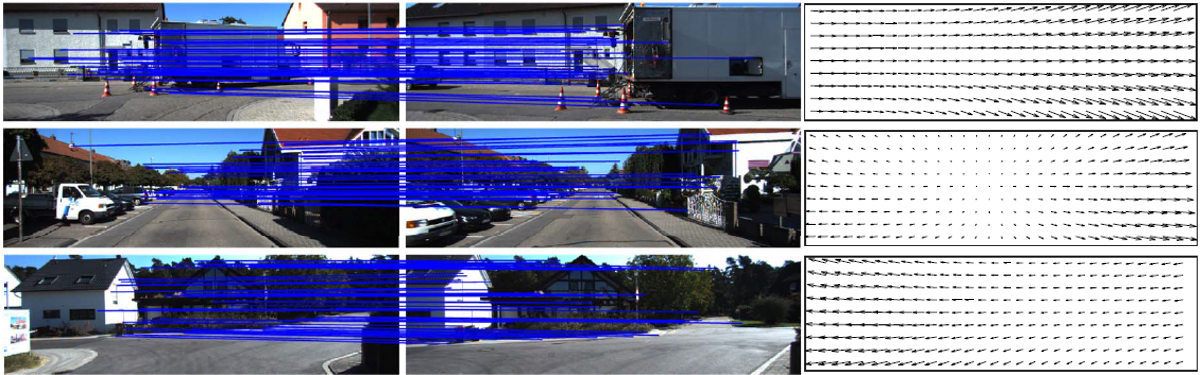
Appearance-Based Loop Closure Detection via Locality-Driven Accurate Motion Field Learning
Kaining Zhang, Xingyu Jiang, Jiayi Ma
IEEE Transactions on Intelligent Transportation Systems (TITS), 2021
- This is a more complete version of our IROS 2021. We propose a more reasonable loss to revise the motion field, and decrease the time complexity of the algorithm from O(N^3) to O(N). Meanwhile, experiments are conducted on more challenging datasets.
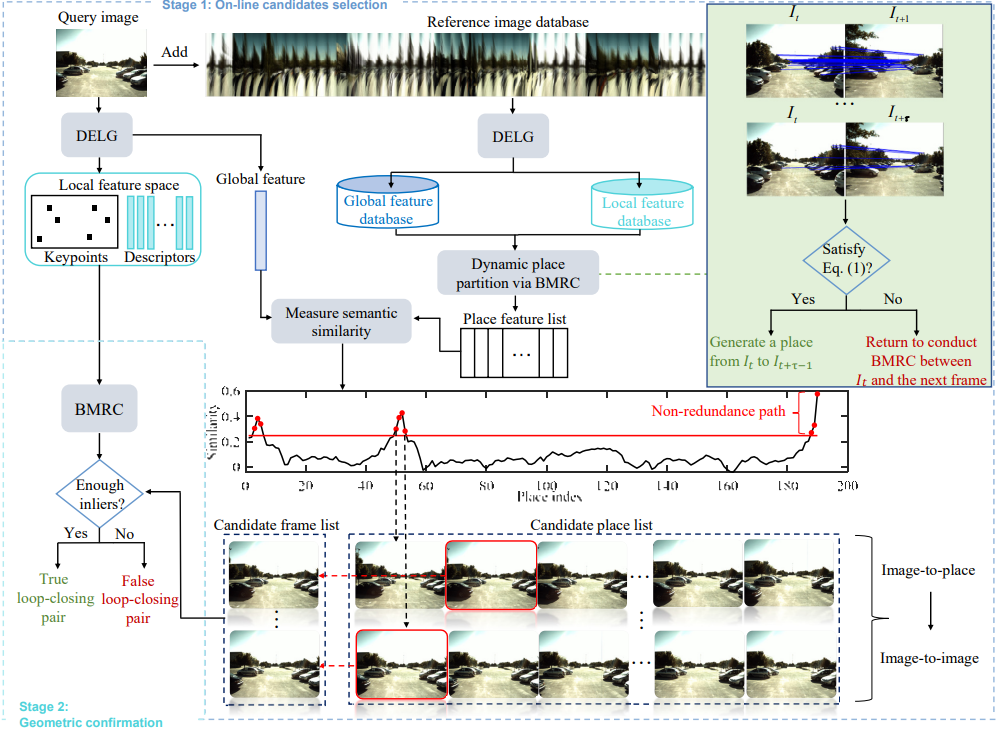
Appearance-based Loop Closure Detection via Bidirectional Manifold Representation Consensus
Kaining Zhang, Zizhuo Li, Jiayi Ma
IEEE International Conference on Robotics and Automation (ICRA), 2021
- We attempt to address loop closure detection (LCD) from the semantic aspect to the geometric one. Based on this idea, the proposed LCD system can achieve satisfying results.
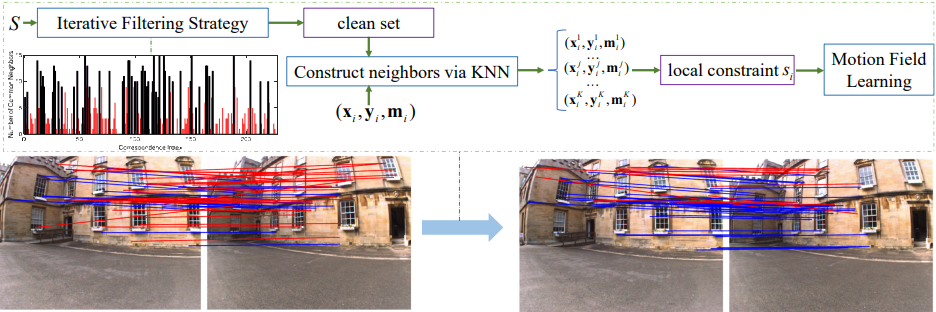
Kaining Zhang, Xingyu Jiang, Xiaoguang Mei, Huabing Zhou, Jiayi Ma
IEEE/RSJ International Conference on Intelligent Robots and Systems (IROS), 2021
- We address feature matching in the perspective of motion field recovery, and add extra constraints to the motion field to make it more suitable for scenes within loop closure detection.